Boost Product Filter & Search aims to optimize the online shopping experience. We have built an effective site search engine and exceptional product filters that merchants can rely on. The app is loved by approximately 14,000 merchants with more than 1,500 five-star reviews on Shopify App Store. When brainstorming about how we can help users even more, we came up with a complete solution to online product discovery. The new Product recommendation feature is our first step in realizing this goal.
Product Recommendation Models In The Boost App
In the upcoming release, we will introduce 8 different recommendation types based on 3 core recommender systems both basic and advanced, both automatic and manual.
- AL/ML-based models (can also use rules to set up)
- Frequently bought together (FBT)
- Related items
- Alternative products
- Complementary products
- Mix of the 2 sub-models
- Statistic-based models:
- Newest arrivals
- Trending products
- Bestsellers
- Most viewed
- Recently viewed
- Manual model:
- Hand-pick products
Read more:
AI/ML-based Product Recommendation Models
We embrace cutting-edge technology of AI/ML in FBT and Related items to auto-detect and suggest products.
Right after you install the new Boost app, the “robot" behind the scene will keep an eye on various data points such as your own store' product data, how your products are combined with each other, how your products are different from each other, and customers' behaviors such as add-to-cart events.
Don't worry about security issues here we apply strict requirements for the data-collecting process. Moreover, we only have “View" permission for all the data so leakage can be said to be impossible. Also, some models don't require historical purchase data so you can set up and run right away.

The new “knowledge" of your store will then be consolidated with other stores of the same segments, all done by machinery. Therefore, our AI models can be tailor-made for distinct eCommerce verticals. This makes the models more efficient at recommending the proper items for each industry.
Additionally, ML algorithms seamlessly train our AI recommendation system with the latest data. This ensures the recommended products are updated frequently, every 7 days.
Although everything is operated on the fly, there is still room for customization with the human-in-the-loop process. Human-centered AI allows our AI recommendation models can be flexibly tailored for your store.
That’s basically how our AI/ML recommender system work.
Besides using AI/ML, you can also set up rule-based recommendations for these types. There are various product attributes to create rules such as metafield, collection, tag, etc.
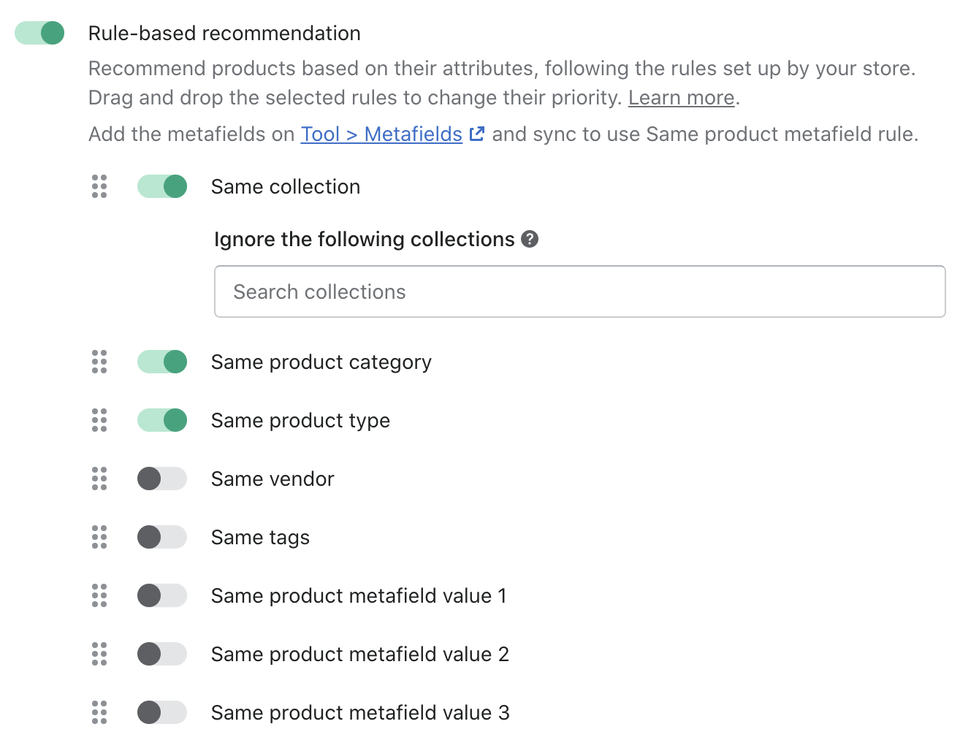
Frequently bought together vs Complementary products
Looking at AI/ML-based recommendation types
- Frequently bought together (FBT)
- Related items
- Alternative products
- Complementary products
- Mix of the 2 sub-models
You might be confused between FBT and Complementary products - the sub-model of Related items (or at least I did when the engineering team first introduced the new feature to me 😅).
So the difference is the data source for a recommendation. FBT items are generated based on items added to the customer’s cart. On the other hand, Complementary products are add-ons of the main product. This means the sub-model uses product data to recommend Complementary products. These products usually add value to the target product or complete the package.
Statistic-based Product Recommendation Models
Recommendation types using statistics are pretty straightforward. We just want to add a small note regarding the timespan that the statistics are renewed in each type.
Recommendation Types |
Time range |
Newest arrivals |
Real-time Based on “Add products" and “Publish products" events of your online store |
Trending products |
Within 7 days Note: You can choose between “View" or “Purchase" events of your customers in recommending Trending products |
Bestsellers |
Within 30 days Based on “Purchanse" events of your customers |
Most viewed |
Within 30 days Based on “View" events of your customers |
Recently viewed |
Real-time Based on each browsing session of your customers |
Manual Product Recommendation Model
Besides using our automatic recommender engines, merchants can curate the recommended products themselves. This is helpful when you want to fix the limelight for a particular set of products without changing or updating them.
To curate your own recommended products, go to Recommendation > Manual settings on Boost's dashboard.
These AI/ML models and statistic-based models, due to their automatic learning nature, will continue to update the recommended products. In some cases, this might not be the best approach. For example, if you have some overstocked items that need clearing out, you can hand-pick these products in the Manual settings under the Recommendation menu so they get more attention from customers.
Tip: If you think adding recommended products one by one is too time-consuming, we suggest you structuring your product data based on metafield or tag. You can then use Rule-based models FQT and Related to keep the recommended products unchanged.
4 Webpages That Boost's Product Recommendation Supports
To increase the add-to-cart rate, it's a great idea to recommend suitable products on the customer journey. Therefore, Boost allows merchants to add recommendation widgets on different pages (more pages are coming).
The recommendation types you can use on each page are not the same due to the nature of that page. For example, you can't use the Frequently Bought Together model on Homepage because there is no source product for the model to generate frequently bought together items.
Below are feasible recommendation types on different pages.
Webpages |
Supported Recommendation Types |
Supported Layout |
Homepage |
|
Carousel, Grid |
Collection page |
|
Carousel, Grid |
Product page |
|
Carousel, Grid Note: Frequently bought together & Related items can be displayed as Product Bundles. |
Cart page |
|
Carousel, Grid |
Product Exclusion In Boost's Product Recommendation Feature
The ultimate goal when we built product recommendations features for Boost AI Search & Discovery is to expand the basket size, thereby, increasing average order value. Therefore, products that don't serve these purposes aren't included in the pool for recommendations. To be more specific, the following products are never taken into account by all of our recommender engines.
- Out-of-stock products
- Products that belong to non-fulfilment locations
- Free products (product price is set at $0.00 or products don't have a price)
- Draft products, archived products, etc (any product that is NOT published to an Online Store sales channel)
Why Do You Need Multiple Recommendation Types?
After all, why do we build so many recommendation models? Why should you try the Product recommendation feature of Boost AI Search & Discovery.
Each business has its own need and preference. More options bring merchants a hybrid approach. You can try out and test several types and several positions to see which models offer the highest return. After the upcoming epic release, we will add a dedicated view for the Recommendation feature with lots of insightful metrics in our Analytics dashboard. The reports help you compare widget types, widget placement, and even the recommended products.
Overview of Recommendation analytics that is expected to be released soon.
Currently, hundred of early-bird users have been experiencing the new and advanced Product Recommendation of Boost and left positive feedback. We expect to bring this revenue-boosting feature together with AI-powered Search, upgraded Visual Merchandising, and more. So, install the app now to get the chance to access these features early